
Marta Kwiatkowska
Marta Kwiatkowska
University of Oxford
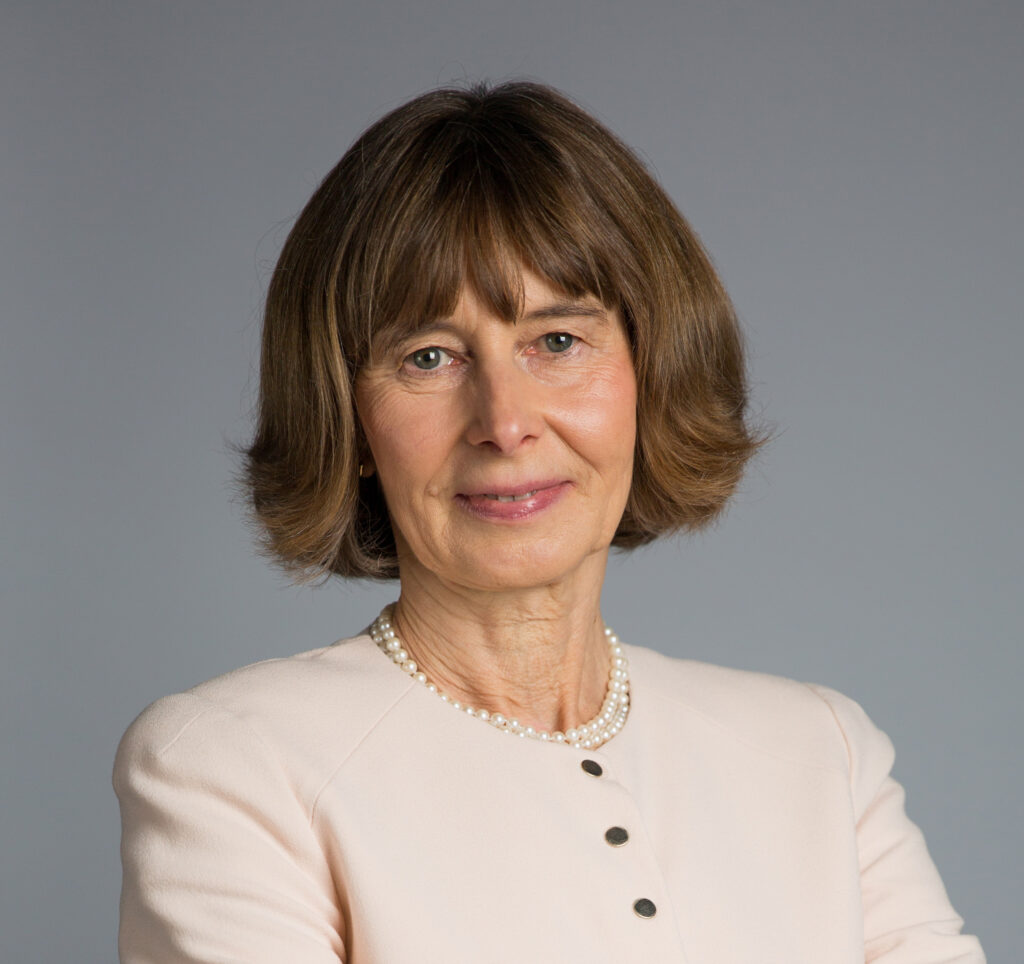
Provable guarantees on robustness of Bayesian networks to causal interventions
Abstract
In many domains, worst-case guarantees on the performance (e.g., prediction accuracy) of a decision function subject to distributional shifts and uncertainty about the environment are crucial. Such shifts can be viewed as interventions on a causal graph, which capture (possibly hypothetical) changes in the data-generating process, whether due to natural reasons or by the action of an adversary. We consider causal Bayesian networks and formally define the interventional robustness problem, a novel model-based notion of robustness for decision functions that measures worst-case performance with respect to a set of interventions that denote changes to parameters and/or causal influences. By relying on a tractable representation of Bayesian networks as a probabilistic circuit, we provide efficient algorithms for computing guaranteed upper and lower bounds on the interventional robustness probabilities. We also develop a method to quantify the robustness of decision functions with respect to credal Bayesian networks, formal parametric models of the environment where uncertainty is expressed through credal sets on the parameters. We implement the methods and provide experimental evidence that the bounding is effective and scalable.
The lecture will be based on the following two articles:
Paper 1: Robustness Guarantees for Credal Bayesian Networks via Constraint Relaxation over Probabilistic Circuits.
Paper 2: Provable Guarantees on the Robustness of Decision Rules to Causal Interventions.
and participants may also find this follow up paper of interest:
Paper 3: Compositional Probabilistic and Causal Inference using Tractable Circuit Models.